Businesses generate and receive a lot of data, which begs to be analyzed. The technology of embedded analytics embraces the latest developments in terms of data mining, processing, visualization, and storage to help the decision-making process.
The very idea of embedded analytics is to make the results as straightforward as possible so that any user or application can use them. In this article, we’ll take a look at 9 embedded analytics tools and how to choose the one that would fit your business.
What Is Embedded Analytics?
Embedded analytics is a workspace where data analysis happens without the need for the user to switch to another app.
Gartner
Embedded analytics is integrated into your business processes and provides you with the necessary business intelligence (BI) for your decision-making. The latest technologies, such as deep learning and artificial intelligence, empower embedded analytics even further to find patterns, connections, and themes in your business data. They also help to enhance the accuracy and speed of the insights.
Embedded Analytics Versions
There are many ways you, as a user of such analytics, can get data and results:
- Data visualization and dashboards (similar to what you get in Google Analytics)
- Interactive and static reports
- Self-service analytics, when you can ask your own question regarding the data in front of you
- Benchmarking
- Mobile reporting
- Visual workflows
Psst!
Do you work for a company that develops embedded analytics tools?
Send us a message and we’ll add your product to our list.
10 Innovative Embedded Analytics Tools
Among the tools that are available (and not counting custom ones that you can develop for your business with the help of HUSPI developers), we think there are seven that stand out the most.
Let’s take a look at them in detail.
Sisense
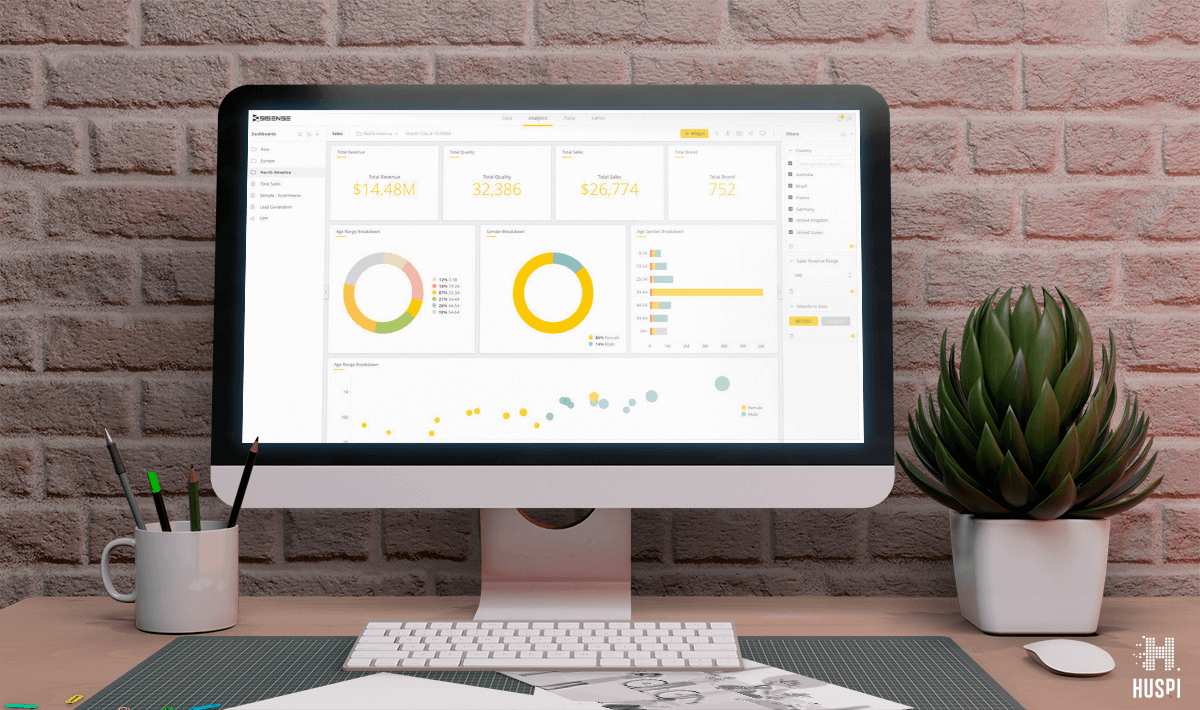
Website: www.sisense.com
Sisense is a powerful BI platform, which provides a single repository for business data and supports information sharing. It is also very user-friendly, which makes it an approachable choice for many businesses.
As an embedded analytics solution for the enterprise world, it offers the chance to avoid choosing between scalability and agility. Sisense offers to optimize data workflows by uniting fragmented data and automatically scaling performance when the data amount increases. This allows for staying on top of the business processes.
Features include:
- Data discovery and visualization
- Pre-built visualization formats such as heatmaps, scatter plots, and others
- Geographical and location analytics & visualization
- Predictive analytics
- ML models support
- Data mining and pattern recognition features
- Integration with R and other statistical packages
Sisense has a free trial available and provides premium consulting and integration services.
You might also be interested: JavaScript Frameworks
QlikView
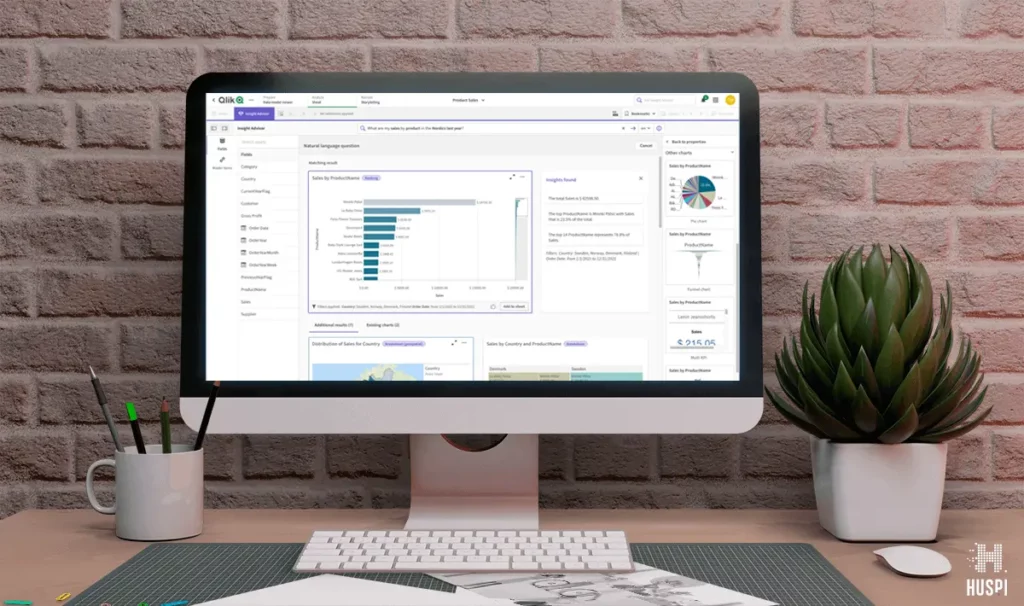
Website: www.qlik.com
QlikView is a business intelligence platform that provides interactive dashboards, data visualizations, and self-service analytics capabilities. It is known for its ease of use and fast implementation.
Here are some of the key advantages of QlikView:
- Fast data processing: QlikView is designed to handle large volumes of data quickly and efficiently. It uses an in-memory data model that allows users to perform real-time data analysis without having to wait for data to be processed.
- Interactive dashboards: QlikView provides interactive dashboards that allow users to explore data in a visually appealing and user-friendly way. This makes it easy for users to identify patterns and trends in their data, and to make informed decisions based on their findings.
- Self-service analytics: QlikView enables users to perform self-service analytics, which means they can explore data and generate insights without needing technical expertise. This can be particularly valuable for small or mid-sized businesses that may not have dedicated data teams.
- Flexible data model: QlikView provides a flexible data model that allows users to easily integrate data from multiple sources. This means that users can access a comprehensive view of their data, which can be useful for making strategic decisions.
- Collaboration: QlikView enables users to collaborate and share insights with other members of their team. This can help to improve communication and ensure that everyone is working from the same data.
- Security: QlikView provides strong security features that ensure that data is protected from unauthorized access. This is particularly important for businesses that deal with sensitive data, such as financial or healthcare data.
QlikView offers a free trial and Qlik Sense® Business Cloud Solution starts at $30/user monthly.
Tableau
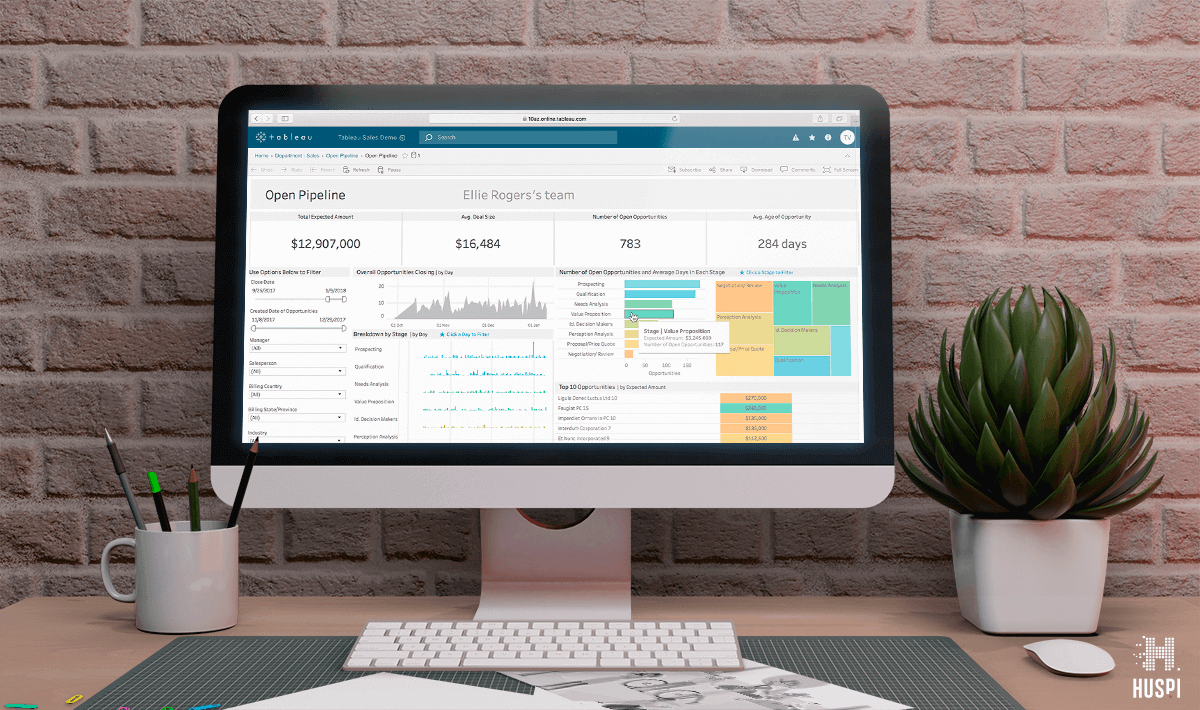
Website: www.tableau.com
Tableau is another powerful player in the market. Tableau can be linked to multiple data sources, which is a great feature when you have data coming from all over. As a result, you can analyze and visualize a more complete picture of what’s going on in your business or department.
Tableau platform has several products you can choose from or combine:
- Tableau Desktop for visual analytics.
- Tableau Prep helps combine, shape, clean, and operationalize the data flow.
- Tableau Server offers collaboration and the ability to share your data and dashboards.
- Tableau Online is basically the Server, but without an actual physical server to maintain.
Tableau’s features include:
- Simple installation
- Interactive dashboards with intuitive design
- Drag ’n’ drop functionality
- Natural language queries
- Powerful data analysis instruments to identify trend lines, cluster data to detect relationships, or see statistical summaries.
- Full analytics journey support – from data preparation to deep analysis to shared insights to drive the business forward.
There is a 14-day free trial available for Tableau’s products or, if you are a student or a teacher, you can enjoy a one-year free trial. There are no entry-level setup fees and the company offers consulting and integration services if necessary.
Toucan Toco
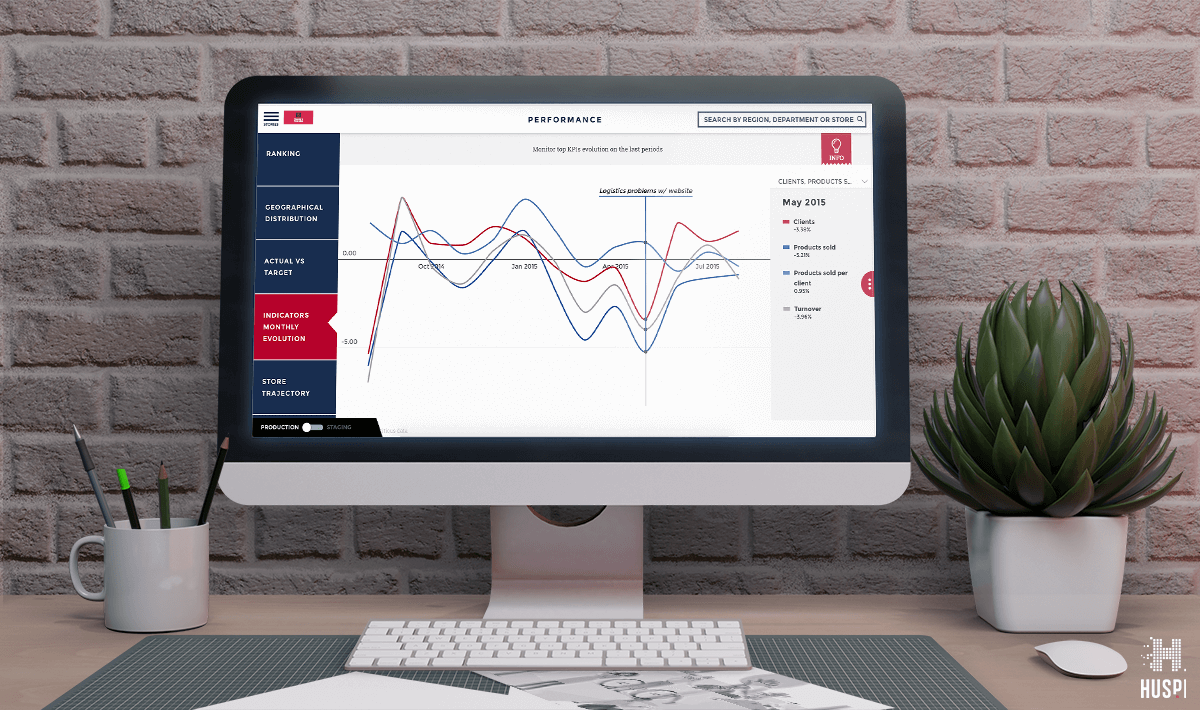
Website: www.toucantoco.com
One of the main attractive characteristics of the Toucan Toco analytics tool is what they say on the homepage: “Toucan makes it easy to connect to any data and deliver insights to everyone in minutes — no Ph.D. required.”
Toucan’s features include:
- Visual design for everything
- Prototype designs (including demo data) and deploy with a click
- Complete white labeling
- A complete library of customizable charts
- Built-in palettes (as well as the opportunity to create personalized ones)
- Modular architecture
- Included connectors for new data sources
- Revolutionary YouPrepTM technology for no-code visual data preparation
- Easy scaling — any data source, any numbers, and any devices
Toucan Toco offers a free demo and guided trial for new users.
Need analytics integrated into your business? Click here
Reveal Embedded Analytics
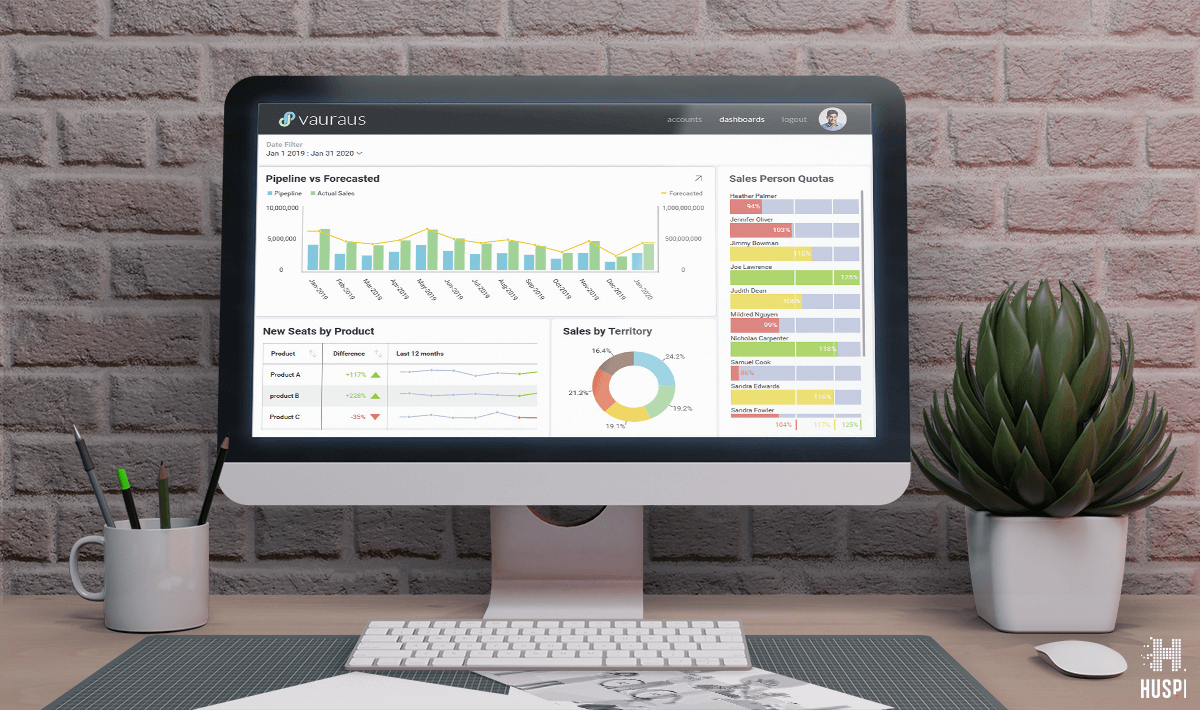
Website: www.revealbi.io
From Reveal’s website: “Cut the time to create and manage dashboards for your end-users and eliminate vendor-side dependencies. Reveal’s responsive dashboards such as marketing and KPI dashboards look beautiful everywhere with touch and smooth scrolling on all screen sizes. Use our powerful APIs to quickly add data analytics capabilities to existing applications with our native web, desktop, iOS, and Android SDKs.”
Reveal’s features include:
- Ability to be deployed or connected to any in-house or cloud-based servers
- Container and microservice architecture for scalability, deployment, and resilience
- Statistical functions for trends identification
- Full Encryption
- Custom actions based on users’ interactions
- Dashboards from one’s application memory with Reveal’s in-memory data source
The price depends on the requirements, but there are several fixed price options with no hidden fees, usage, or user tiers.
SAP Crystal & SAP S/4HANA
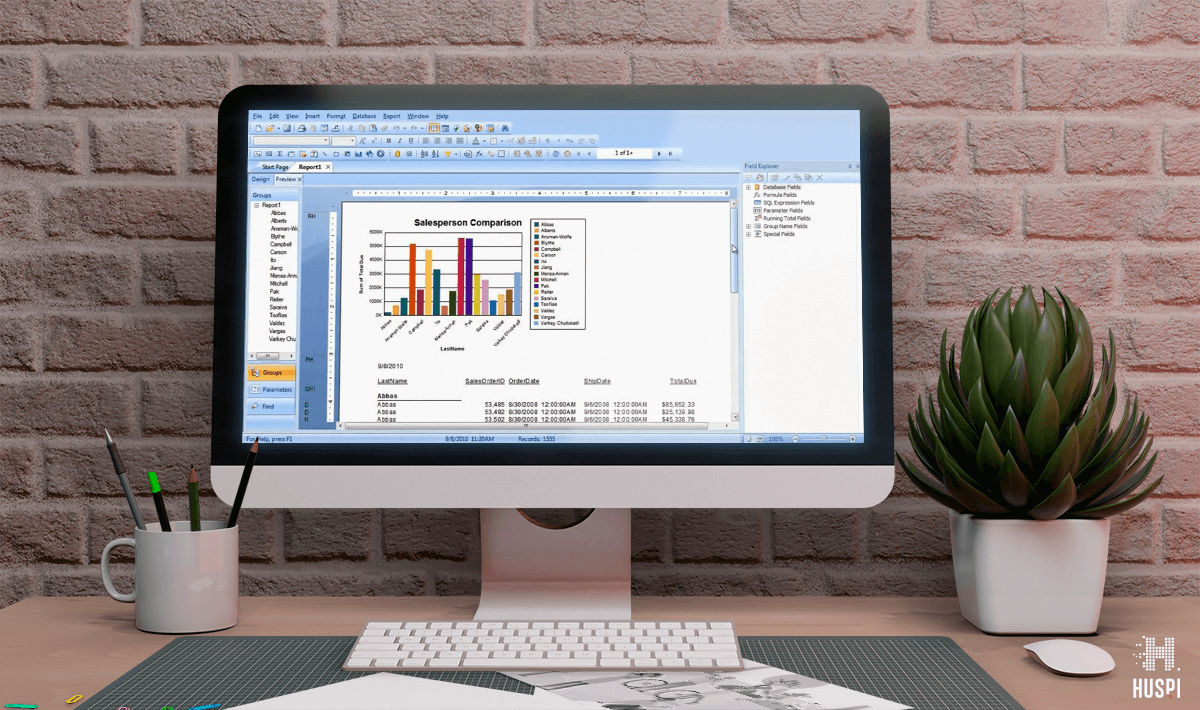
SAP Crystal website: www.crystalreports.com
SAP S/4HANA website: www.sap.com/s4hana
What is embedded analytics in SAP and what is embedded analytics in S4 HANA?
SAP Crystal Reports and SAP Crystal Server are embedded analytics tools designed to help create beautiful, richly formatted, and dynamic reports that combine one or several data sources into interactive, actionable insights.
Since SAP is a large enterprise, its products boast dozens of formats and up to 24 languages. It might not be the most user-friendly instrument from the very start, but it sure is powerful.
SAP BI Platform Features:
- Web interface for administration – the ability to access your data from wherever you are
- Snapshot of and a live connection to external data
- Multi-data source connectivity, making it able to provide real-time operational reports to make day-to-day business decisions
- Numerous options to deliver insights and a clearer overall picture of the business for the decision-makers
- Flexible and customizable report design to make the insights digestible and easy to understand
There is a free trial available as well as consulting/integration services.
SAP has another product that deals with data insights. SAP S/4HANA product provides instant insights on live data without redundancy or latencies. You don’t need ETL (Extract, Transform, Load) process or batch processing as compared to the traditional landscapes that involve various ERP systems.
SAP S/4HANA employs artificial intelligence technology for embedded analytics and key decision management aspects, such as:
- Strategic decision-making (based on historical data)
- Operational decision-making (based on recent business data)
- Tactical decision-making (based on a comparison between current data vs. contextual data from the past)
SAP S/4HANA has a free 14-day trial.
Looker
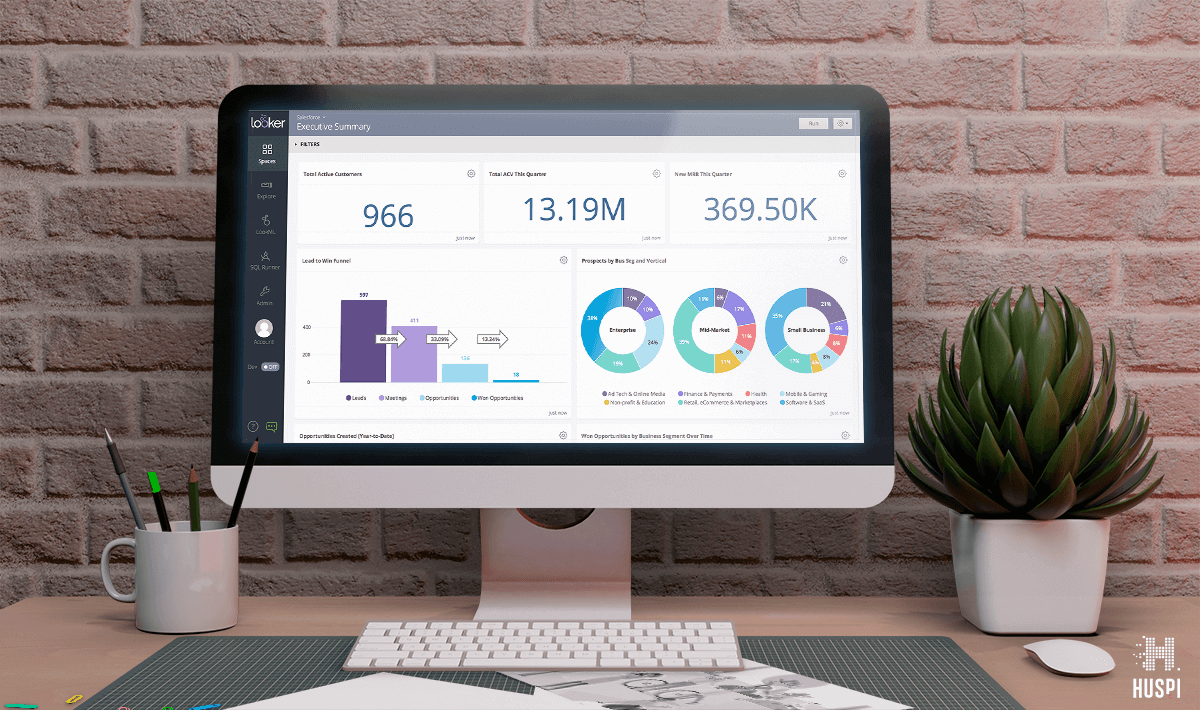
Website: looker.com
Looker has several great features including Business Intelligence and Embedded Analytics & Reporting. Thanks to them, clients can monetize the data they are getting and reduce costs because of an enhanced decision-making process.
Looker welcomes collaboration and features drag-and-drop functionality. It also comes with its own language called LookML, which can be used to create one’s own apps.
In the summer of 2019, it struck a deal with Google Cloud, so now Looker will be a part of the GCP.
We’re very excited to announce that Google has entered into a definitive agreement to acquire Looker, a unified platform for business intelligence, data applications, and embedded analytics. Upon the close of the acquisition, Looker will join Google Cloud.
Thomas Kurian, CEO of Google Cloud
Features:
- Pre-built visualization formats such as heatmaps, scatter plots, and others.
- Geographical location analytics and visualization
- Machine learning models support
Microsoft Power BI
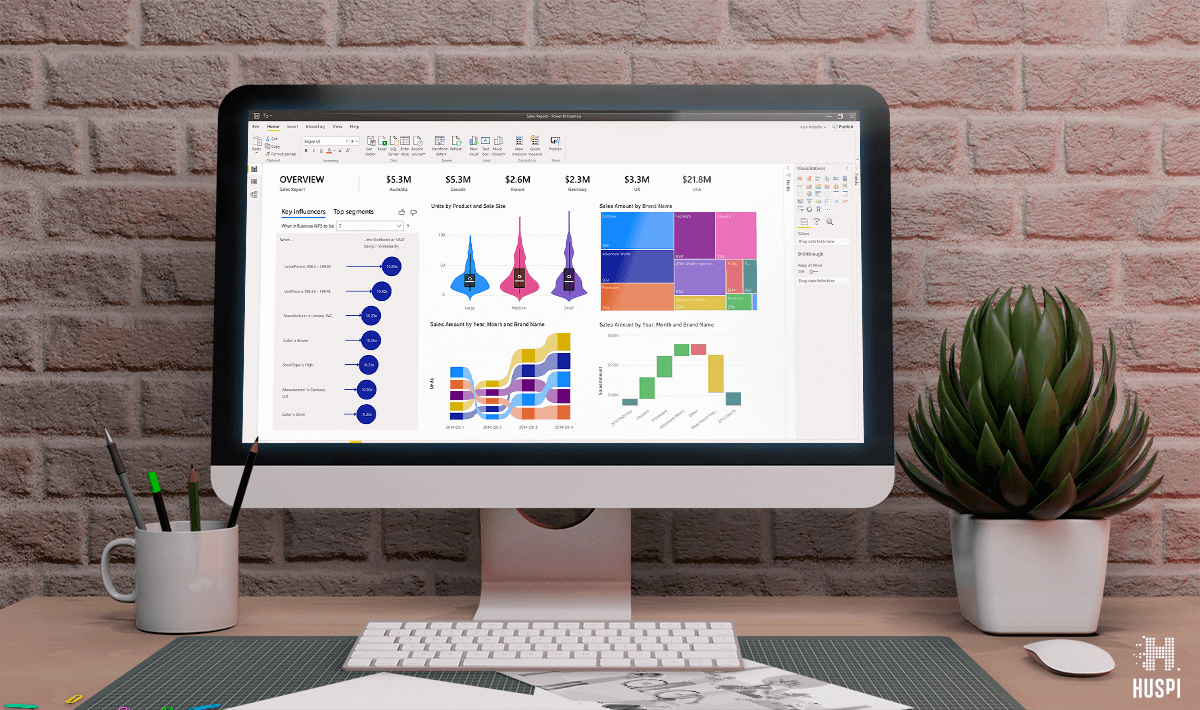
Website: powerbi.microsoft.com
Power BI by Microsoft is a business analytics tool, which helps you visualize your data and share insights with your team members and collaborators. You can also embed these insights into your app or website.
Power BI has the feature of multiple data sources – up to hundreds – that bring the data to life with live dashboards and reports.
HUSPI has experience with integrating Microsoft Power BI for one of our clients – MIRS Corporation. The task before us was to unify several large e-commerce databases into one wholesome ecosystem, which allowed us to streamline decision-making processes given a large amount of information.
Key Power BI features:
- Simple and free initial setup
- Connectivity with Excel spreadsheets, cloud services, on-premise databases, and streaming data
- Real-time updates for dashboards
- Natural language processing (NLP) for data visualization tools
- A collaborative framework that promotes sharing reports and datasets
- Integration using REST API
- Publish-to-web feature for publishing data directly from the service
Since it’s a Microsoft product, Power BI doesn’t have a standalone app for Macs and iPhones, just Windows-based or in the cloud.
Oracle BI Foundation Suite
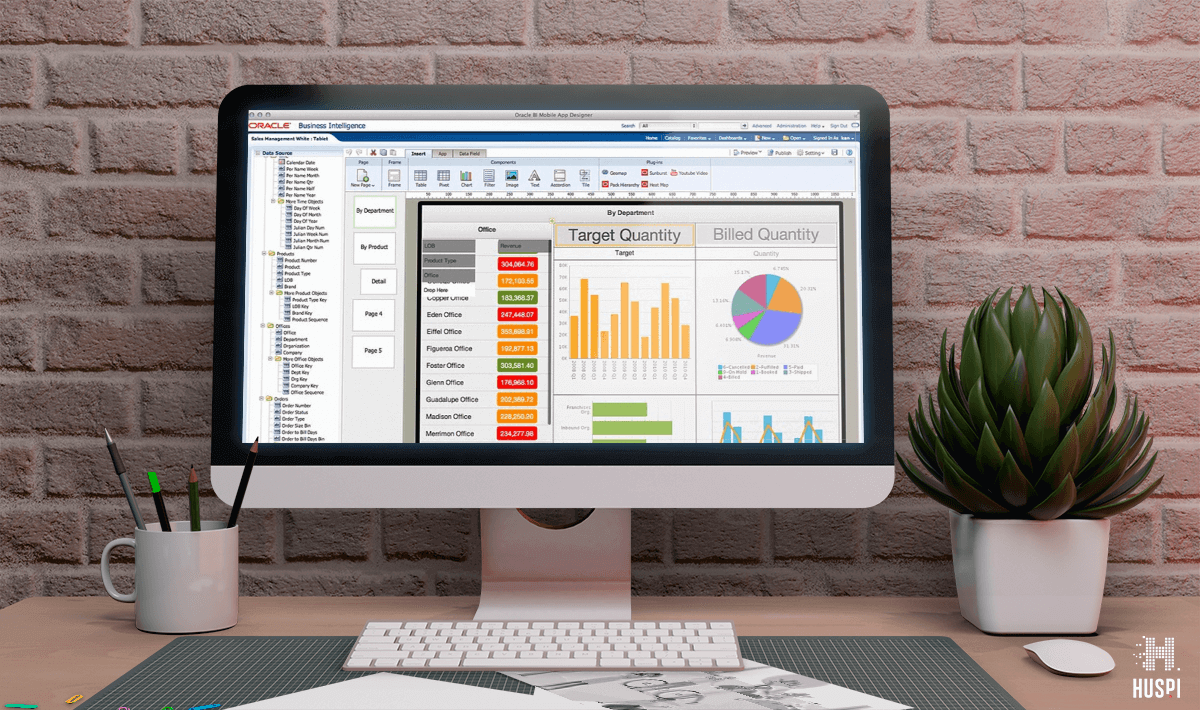
Website: www.oracle.com/bi-foundation
Powerful Oracle Corporation has a great enterprise tool for embedded analytics and business intelligence called the Oracle Business Intelligence (BI) Foundation Suite. It’s a bundle of integrated tools that help with decision-making.
The BI Foundation Suite also enables Oracle Exalytics (a system for in-memory analytics for business intelligence and planning applications) as well as Oracle Real-Time Decisions (a platform that combines predictive analytics and rules for real-time enterprise decision management.)
Oracle BI Foundation key features:
- Ad-hoc query and analysis
- Comprehensive data visualization suite
- Enterprise reporting of high-quality
- Scalable server for forward-looking analysis
- Powerful mobile app
- Integrated strategy management and scorecard
Bitrix24
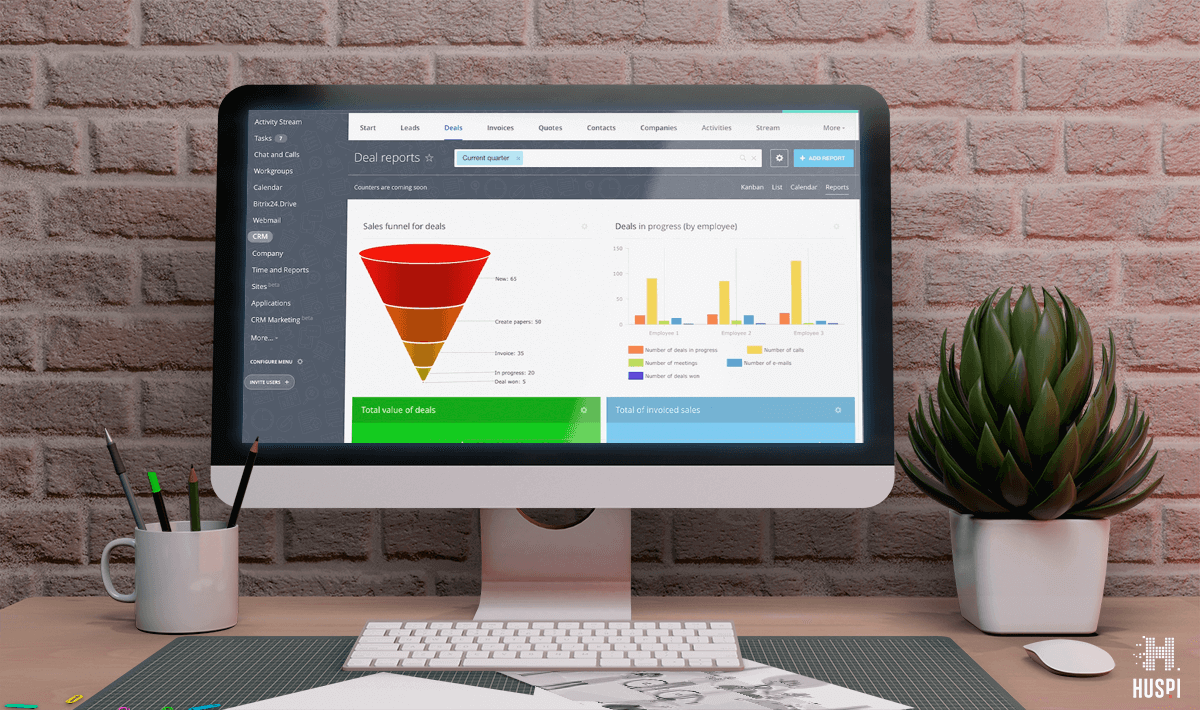
Website: www.bitrix24.com
Bitrix24 is a business management software that is 100% free. It is available both in the cloud as well as software that can be installed on the server and be ready to use within 30 seconds.
While it’s more of a management instrument rather than pure embedded analytics, we included this instrument in our list because, besides analytics, it also offers over 30 other tools available for free. Bitrix24 is a great tool for small and medium businesses.
It comes with default and custom reports right in the CRM, which allow analyzing activities, sales, conversion rates, deal pipelines, and others. You can create your own report template or use one of the available popular report templates.
Bitrix24 also has built-in marketing reports and sales intelligence, which gets data from Google AdWords, Facebook Ads, and many other channels.
Features include:
- All-in-one stop for business management and intelligence
- Intuitive dashboard
- Performance metrics
- Marketing & Sales Intelligence
- Report templates as well as the ability to create custom reports
- Cloud or self-hosted options
- Mobile apps for iOS and Android
- Available APIs for further integration
How To Choose an Embedded Analytics Solution?
According to the State of Embedded Analytics Report, released by Business Intelligence and Analytics company Logi Analytics, there are several key areas the embedded analytics ventures have to concentrate on in order to stay afloat and supply relevant features to their clients. These segments include:
- Artificial Intelligence implementation;
- Forecasting analysis;
- Natural language processing;
- Business processes navigation;
- Database reverse entry.
To understand what needs to be done as a priority, you need to identify major business challenges the customers face. Once you’re done with that part, the process of tracking down the technologies will be much simpler.
Don’t run after each of the state-of-the-art apps when you don’t see the real need for it. If you are a small or medium business, it is cost-efficient (not to mention faster) to use embedded analytics instruments such as Bitrix24 or Power BI by Microsoft that offer user-friendly collaboration. However, if you’re an enterprise, it would make more sense to go with SAP or Oracle.
In experts’ opinion, the first thing where everything has to start in this case is predictive analytics. Using the vast volume of previously collected data and employment of Machine Learning algorithms to process this data will allow the detection of the business problems that the people will be able to solve.
There are also issues that businesses will encounter no matter in which industry they work: equipment sometimes fails, business processes may be interrupted, and so on. Solving such problems will improve the company’s key performance indicators and allow building long-term strategic plans to accomplish.
How To Activate Embedded Analytics?
Considering the high development and integration cost for embedded analytics for the enterprise, this isn’t a decision that should be rushed (startups and SMBs have a simpler decision to make usually.)
We advise you to work with business consultants and audit the processes in order to make sure your choice of analytics solution will pay off well. HUSPI has business consultants with a Ph.D. in computer sciences as well as hands-on experience in software development and integration. Send us a message via the contact form to discuss your enterprise needs.
Before the enterprises start integrating progressive attributes into the in-built analytical products, the experts recommend making sure the company is ready for the upcoming changes.
Usually, when customers come up with the idea for applications, they deliver a range of requirements. As soon as the solution is released, the clients are likely to present an additional list of requirements that haven’t been stipulated in the first place. Therefore, the customers want to be able to improve the application after it is finally produced. Providing them with the capability to participate in the app development and enhancement process will be a remarkable achievement for the entire software development industry.
The vendor’s development teams can theoretically declare that they can generate the application one hundred percent but it is highly unlikely that it will really happen or at least it will be done within a reasonable period of time. However, if the developers produce about 60 percent of the application and the rest 40 percent are built by the citizen developers who, obviously, have better insight regarding the client’s anticipations, the product will be delivered not only quicker but will provide the customer with better results.
It is presumed that AI-driven forecasting solutions are a proper tool to engage more users and customers beyond the major development ecosystems into the product life cycle. However, this is not the only way to accomplish that.
Indeed, Artificial Intelligence is an effective instrument to make aware of the people with the possibility of creating extra fragments of the applications, especially when they are completely unfamiliar with this job. Nevertheless, other methods to achieve the same goals comprise streamlined experiences and customization tools to fine-tune the reports, information, and forecasting analytics.
The experts say that due to the huge volume of computing efforts needed and the knowledge shortage regarding Artificial Intelligence, more conventional approaches are required.
Certainly, for large-scale businesses that are capable of sourcing a contractor to launch an extensive cloud-based Artificial Intelligence application, this is not a big deal. At the same time, there are lots of companies that can hardly afford that, and the question is how these companies can explore the advantages of the latest computing approaches.
If implemented correctly, embedded analytics can improve business processes and streamline them. They also simplify decision-making thanks to all the AI/ML features many of the tools mentioned above have.
Need to discuss your business requirements for an embedded analytics solution?
Wondering about time-to-value?
Request a no-obligation discovery call and receive a preliminary estimate tailored to your KPIs.